DiTFastAttn: Attention Compression for Diffusion Transformer Models
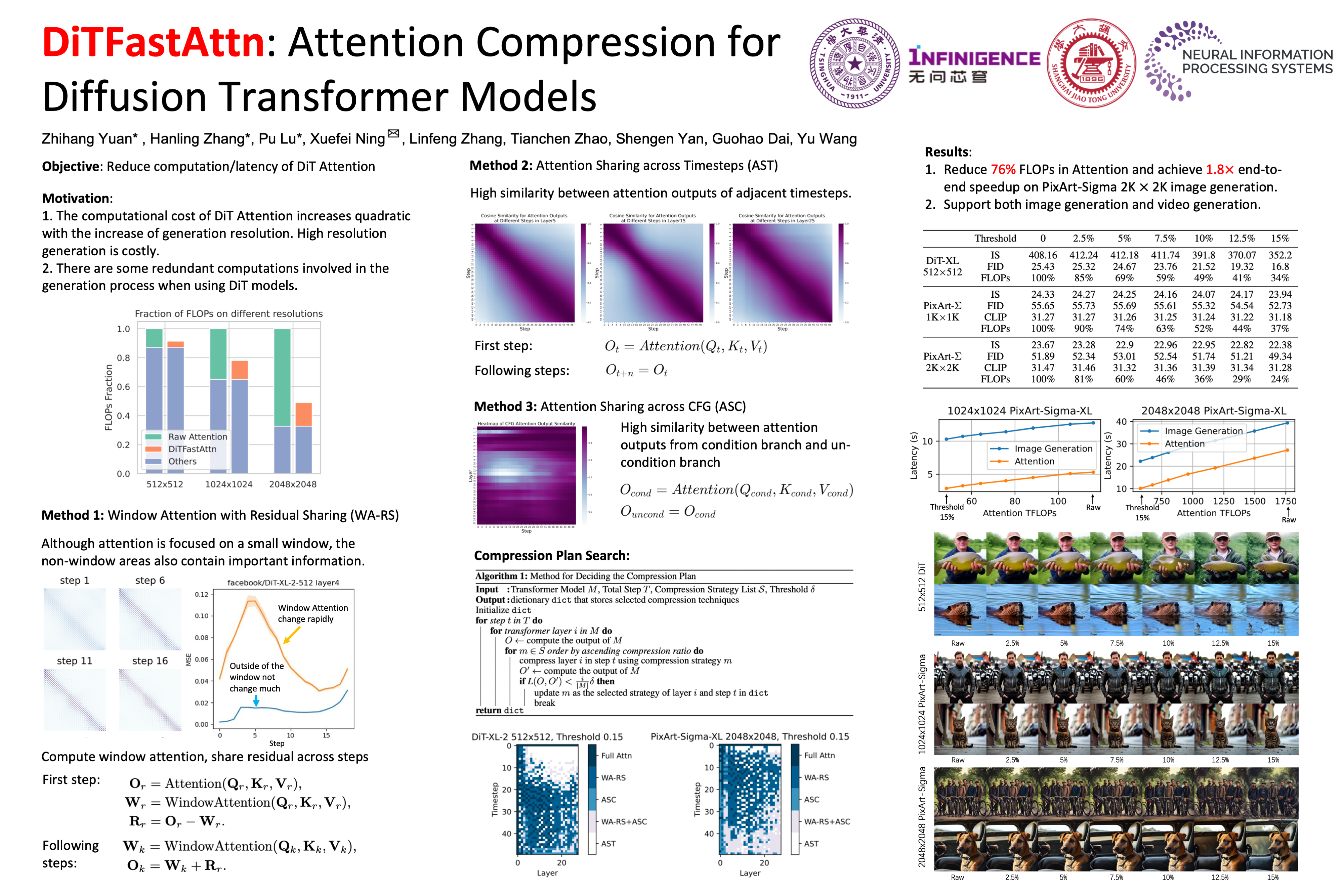
Abstract
Diffusion Transformers (DiT) excel at image and video generation but face computational challenges due to the quadratic complexity of self-attention operators. We propose DiTFastAttn, a post-training compression method to alleviate the computational bottleneck of DiT.We identify three key redundancies in the attention computation during DiT inference: (1) spatial redundancy, where many attention heads focus on local information; (2) temporal redundancy, with high similarity between the attention outputs of neighboring steps; (3) conditional redundancy, where conditional and unconditional inferences exhibit significant similarity. We propose three techniques to reduce these redundancies: (1) $\textit{Window Attention with Residual Sharing}$ to reduce spatial redundancy; (2) $\textit{Attention Sharing across Timesteps}$ to exploit the similarity between steps; (3) $\textit{Attention Sharing across CFG}$ to skip redundant computations during conditional generation.