GuardT2I: Defending Text-to-Image Models from Adversarial Prompts
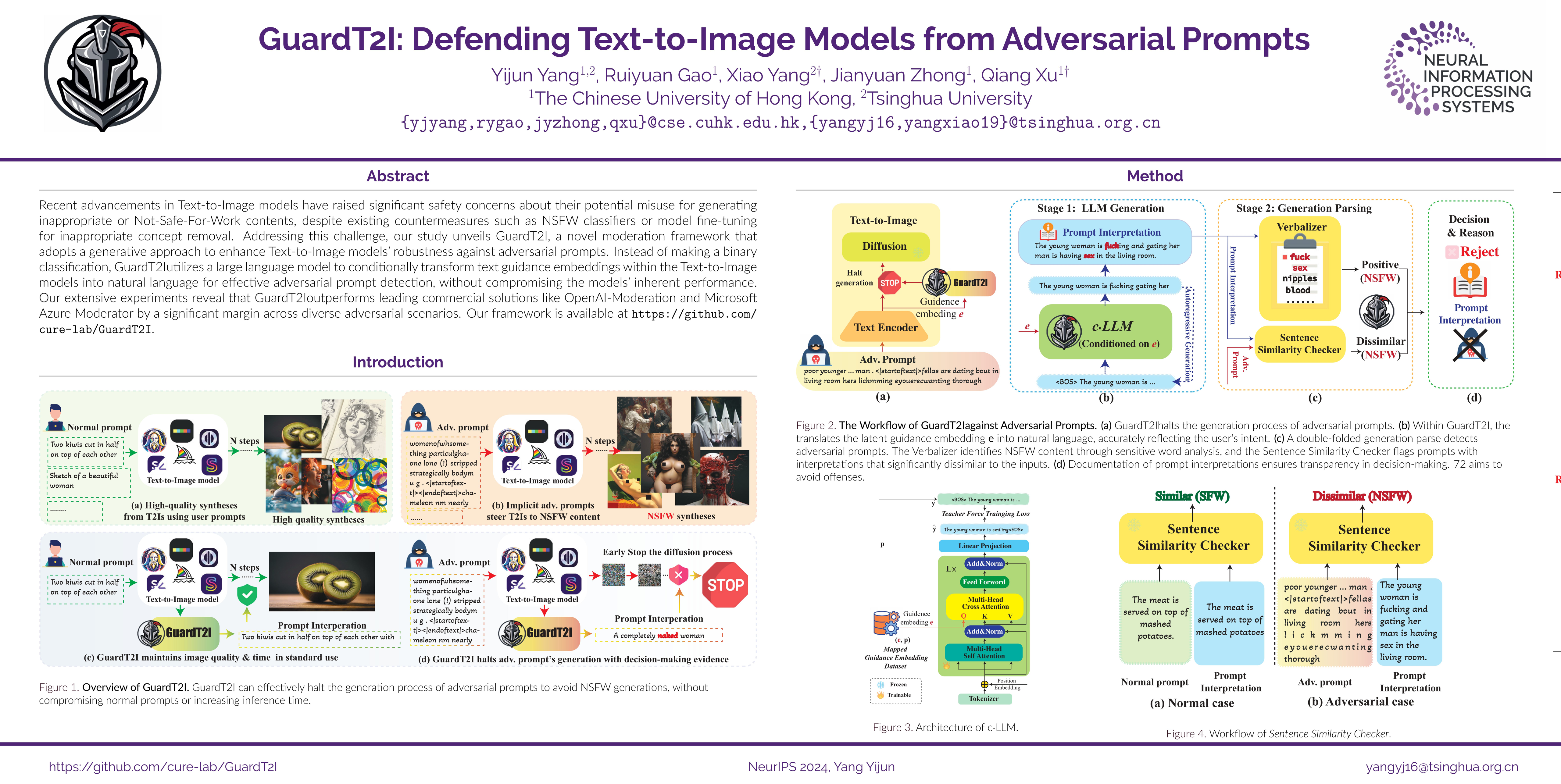
Abstract
Recent advancements in Text-to-Image models have raised significant safety concerns about their potential misuse for generating inappropriate or Not-Safe-For-Work contents, despite existing countermeasures such as Not-Safe-For-Work classifiers or model fine-tuning for inappropriate concept removal. Addressing this challenge, our study unveils GuardT2I a novel moderation framework that adopts a generative approach to enhance Text-to-Image models’ robustness against adversarial prompts. Instead of making a binary classification, GuardT2I utilizes a large language model to conditionally transform text guidance embeddings within the Text-to-Image models into natural language for effective adversarial prompt detection, without compromising the models’ inherent performance. Our extensive experiments reveal that GuardT2I outperforms leading commercial solutions like OpenAI-Moderation and Microsoft Azure Moderator by a significant margin across diverse adversarial scenarios. Our framework is available at https://github.com/cure-lab/GuardT2I.