Association Pattern-aware Fusion for Biological Entity Relationship Prediction
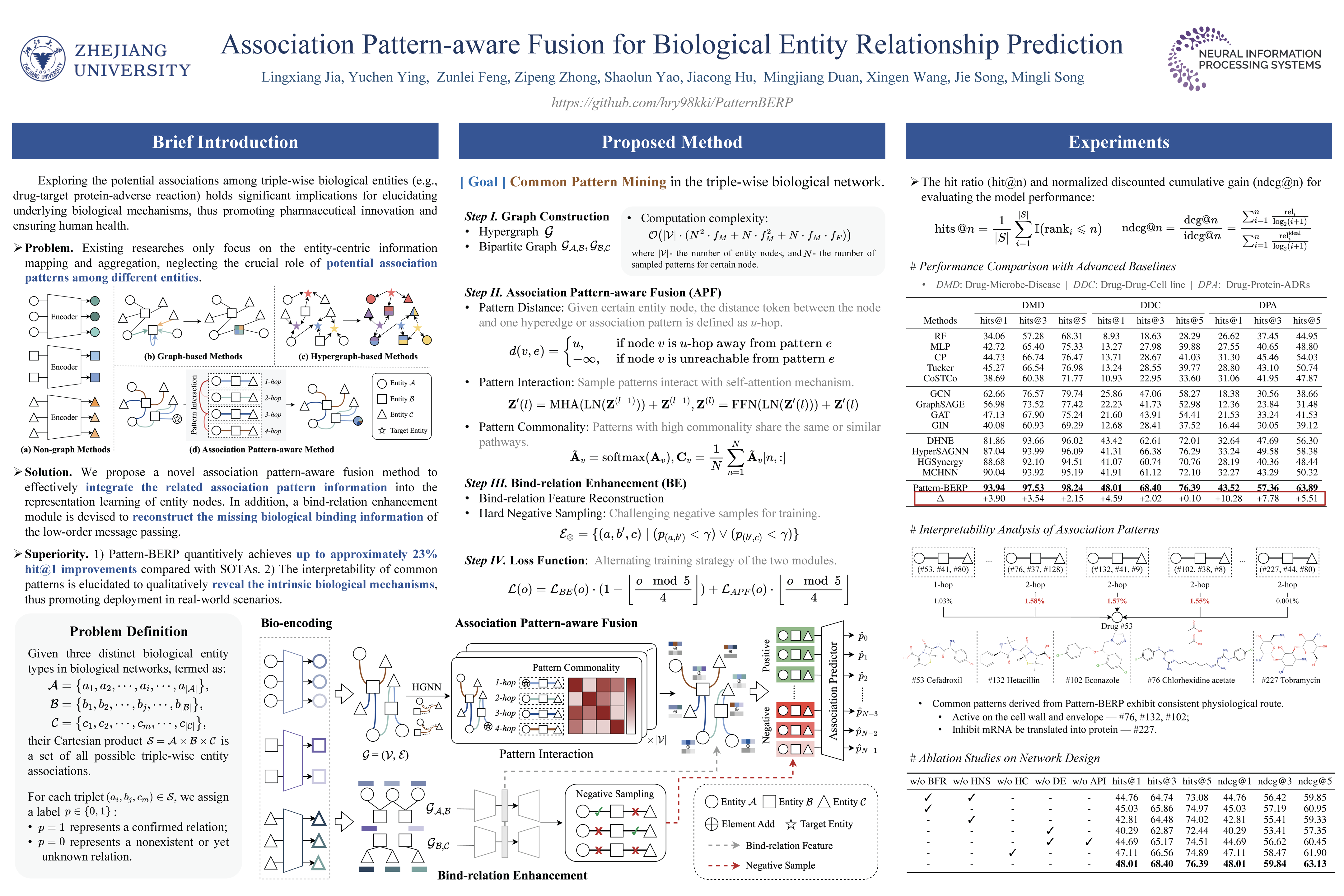
Abstract
Deep learning-based methods significantly advance the exploration of associations among triple-wise biological entities (e.g., drug-target protein-adverse reaction), thereby facilitating drug discovery and safeguarding human health. However, existing researches only focus on entity-centric information mapping and aggregation, neglecting the crucial role of potential association patterns among different entities. To address the above limitation, we propose a novel association pattern-aware fusion method for biological entity relationship prediction, which effectively integrates the related association pattern information into entity representation learning. Additionally, to enhance the missing information of the low-order message passing, we devise a bind-relation module that considers the strong bind of low-order entity associations. Extensive experiments conducted on three biological datasets quantitatively demonstrate that the proposed method achieves about 4%-23% hit@1 improvements compared with state-of-the-art baselines. Furthermore, the interpretability of association patterns is elucidated in detail, thus revealing the intrinsic biological mechanisms and promoting it to be deployed in real-world scenarios. Our data and code are available at https://github.com/hry98kki/PatternBERP.