Density-based User Representation using Gaussian Process Regression for Multi-interest Personalized Retrieval
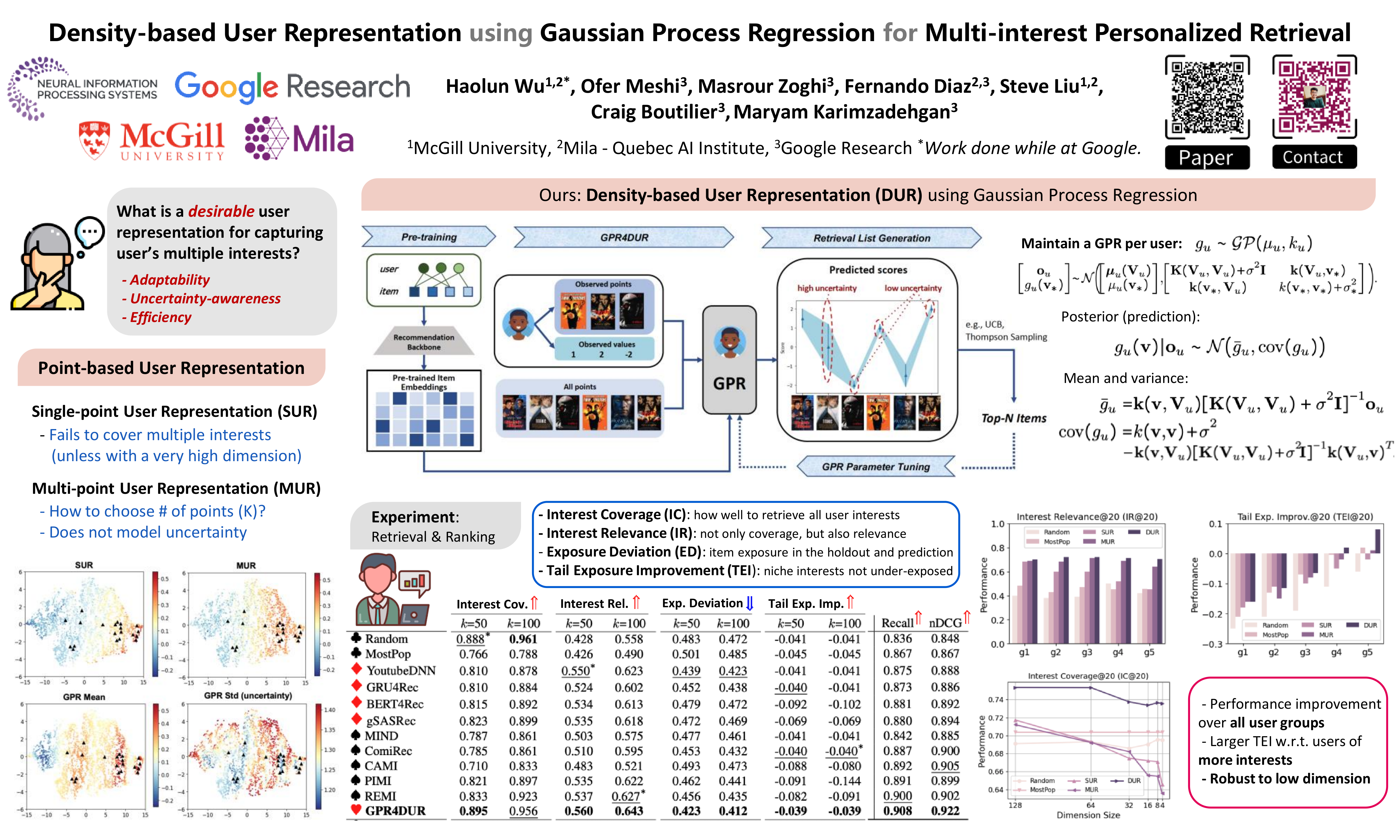
Abstract
Accurate modeling of the diverse and dynamic interests of users remains a significant challenge in the design of personalized recommender systems. Existing user modeling methods, like single-point and multi-point representations, have limitations w.r.t.\ accuracy, diversity, and adaptability. To overcome these deficiencies, we introduce density-based user representations (DURs), a novel method that leverages Gaussian process regression (GPR) for effective multi-interest recommendation and retrieval. Our approach, GPR4DUR, exploits DURs to capture user interest variability without manual tuning, incorporates uncertainty-awareness, and scales well to large numbers of users. Experiments using real-world offline datasets confirm the adaptability and efficiency of GPR4DUR, while online experiments with simulated users demonstrate its ability to address the exploration-exploitation trade-off by effectively utilizing model uncertainty.