On the Identifiability of Hybrid Deep Generative Models: Meta-Learning as a Solution
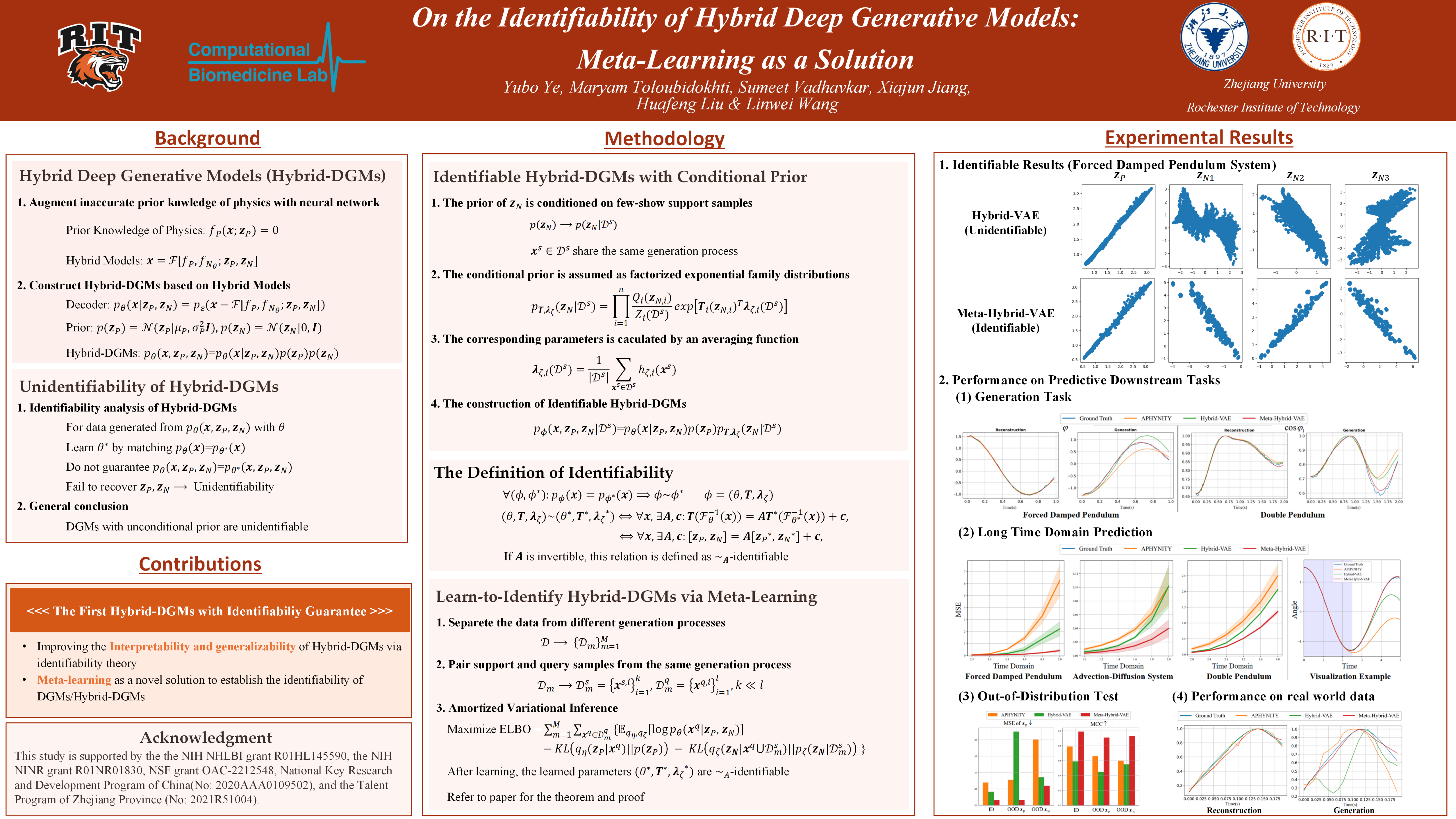
Abstract
The interest in leveraging physics-based inductive bias in deep learning has resulted in recent development of hybrid deep generative models (hybrid-DGMs) that integrates known physics-based mathematical expressions in neural generative models. To identify these hybrid-DGMs requires inferring parameters of the physics-based component along with their neural component. The identifiability of these hybrid-DGMs, however, has not yet been theoretically probed or established. How does the existing theory of the un-identifiability of general DGMs apply to hybrid-DGMs? What may be an effective approach to consutrct a hybrid-DGM with theoretically-proven identifiability? This paper provides the first theoretical probe into the identifiability of hybrid-DGMs, and present meta-learning as a novel solution to construct identifiable hybrid-DGMs. On synthetic and real-data benchmarks, we provide strong empirical evidence for the un-identifiability of existing hybrid-DGMs using unconditional priors, and strong identifiability results of the presented meta-formulations of hybrid-DGMs.