Single Image Reflection Separation via Dual-Stream Interactive Transformers
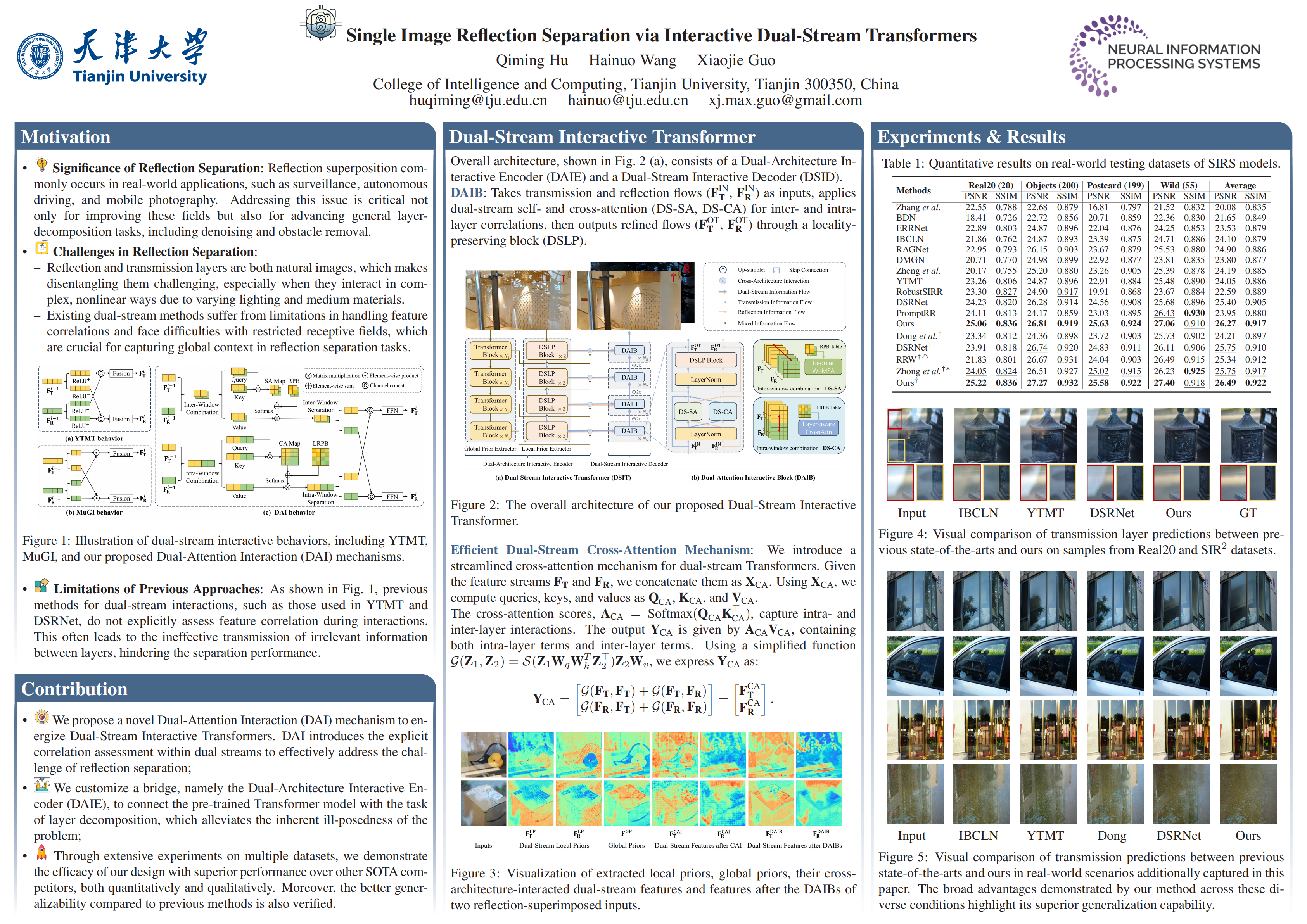
Abstract
Despite satisfactory results on ``easy'' cases of single image reflection separation, prior dual-stream methods still suffer from considerable performance degradation when facing complex ones, i.e, the transmission layer is densely entangled with the reflection having a wide distribution of spatial intensity. The main reasons come from the lack of concern on the feature correlation during interaction, and the limited receptive field. To remedy these deficiencies, this paper presents a Dual-Stream Interactive Transformer (DSIT) design. Specifically, we devise a dual-attention interactive structure that embraces a dual-stream self-attention and a layer-aware dual-stream cross-attention mechanism to simultaneously capture intra-layer and inter-layer feature correlations. Meanwhile, the introduction of attention mechanisms can also mitigate the receptive field limitation. We modulate single-stream pre-trained Transformer embeddings with dual-stream convolutional features through cross-architecture interactions to provide richer semantic priors, thereby further relieving the ill-posedness of the problem. Extensive experimental results reveal the merits of the proposed DSIT over other state-of-the-art alternatives. Our code is publicly available at https://github.com/mingcv/DSIT.