FineCLIP: Self-distilled Region-based CLIP for Better Fine-grained Understanding
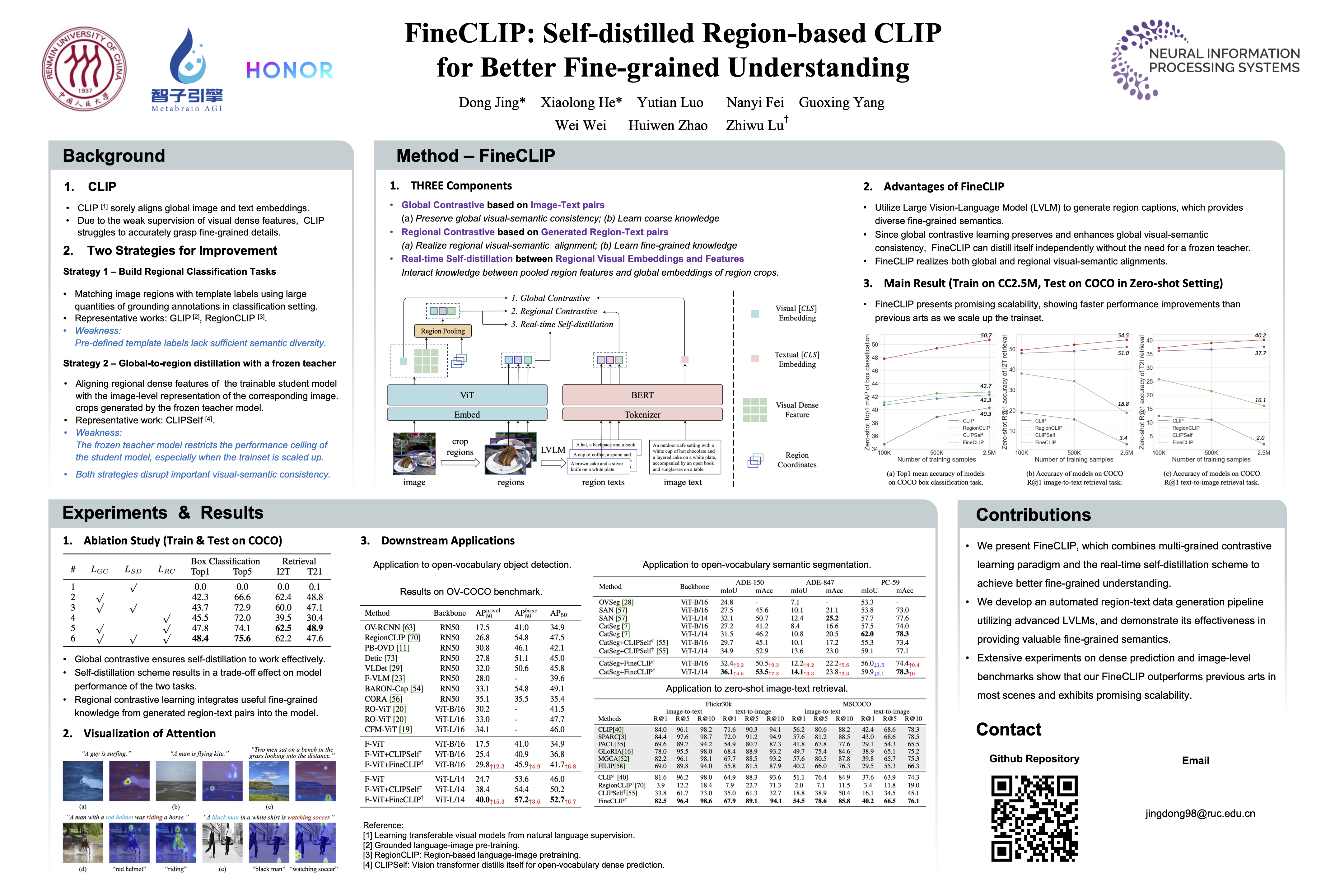
Abstract
Contrastive Language-Image Pre-training (CLIP) achieves impressive performance on tasks like image classification and image-text retrieval by learning on large-scale image-text datasets. However, CLIP struggles with dense prediction tasks due to the poor grasp of the fine-grained details. Although existing works pay attention to this issue, they achieve limited improvements and usually sacrifice the important visual-semantic consistency. To overcome these limitations, we propose FineCLIP, which keeps the global contrastive learning to preserve the visual-semantic consistency and further enhances the fine-grained understanding through two innovations: 1) A real-time self-distillation scheme that facilitates the transfer of representation capability from global to local features. 2) A semantically-rich regional contrastive learning paradigm with generated region-text pairs, boosting the local representation capabilities with abundant fine-grained knowledge. Both cooperate to fully leverage diverse semantics and multi-grained complementary information.To validate the superiority of our FineCLIP and the rationality of each design, we conduct extensive experiments on challenging dense prediction and image-level tasks. All the observations demonstrate the effectiveness of FineCLIP.