EM Distillation for One-step Diffusion Models
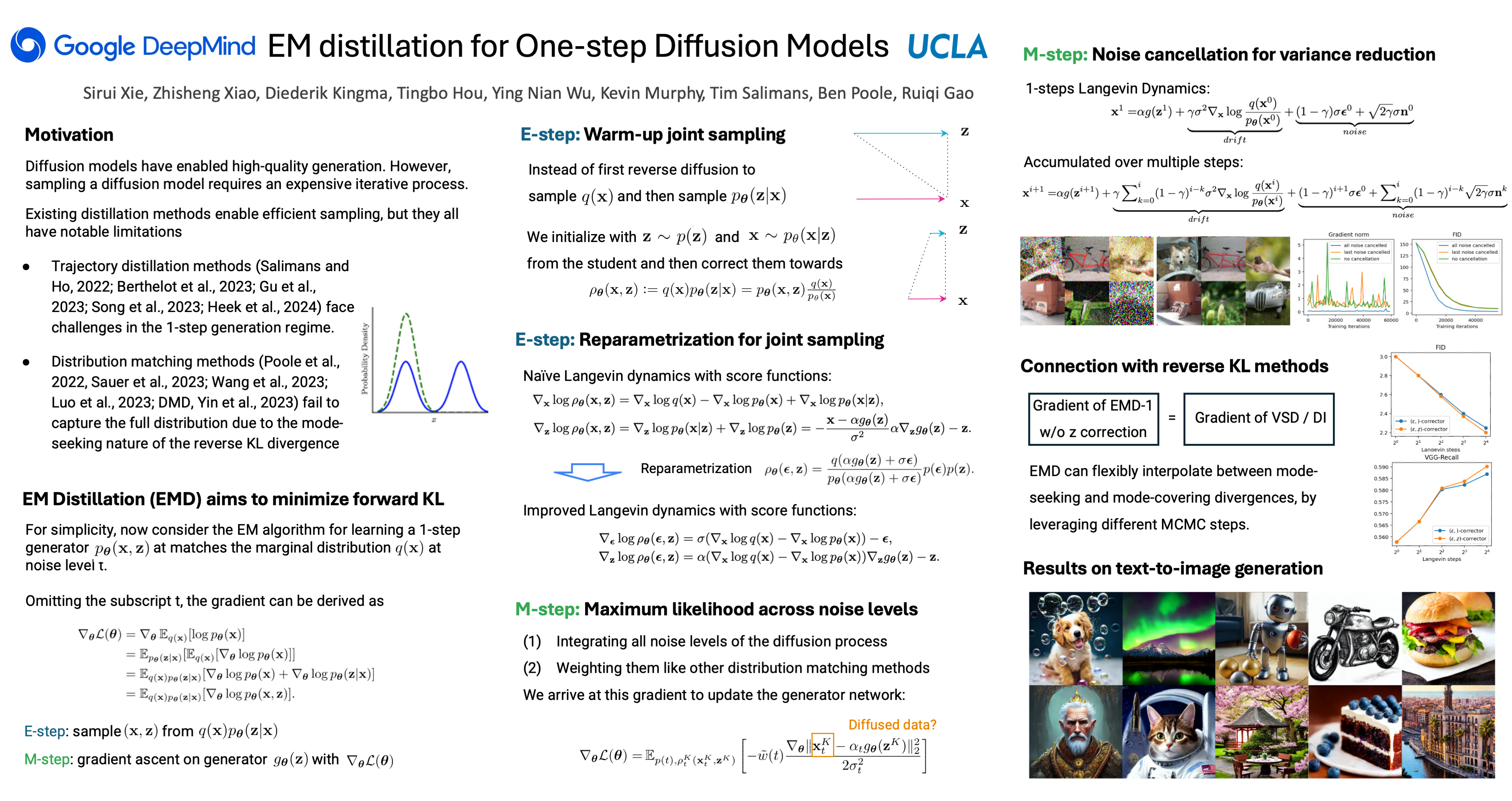
Abstract
While diffusion models can learn complex distributions, sampling requires a computationally expensive iterative process. Existing distillation methods enable efficient sampling, but have notable limitations, such as performance degradation with very few sampling steps, reliance on training data access, or mode-seeking optimization that may fail to capture the full distribution. We propose EM Distillation (EMD), a maximum likelihood-based approach that distills a diffusion model to a one-step generator model with minimal loss of perceptual quality. Our approach is derived through the lens of Expectation-Maximization (EM), where the generator parameters are updated using samples from the joint distribution of the diffusion teacher prior and inferred generator latents. We develop a reparametrized sampling scheme and a noise cancellation technique that together stabilizes the distillation process. We further reveal an interesting connection of our method with existing methods that minimize mode-seeking KL. EMD outperforms existing one-step generative methods in terms of FID scores on ImageNet-64 and ImageNet-128, and compares favorably with prior work on distilling text-to-image diffusion models.