On the Expressive Power of Tree-Structured Probabilistic Circuits
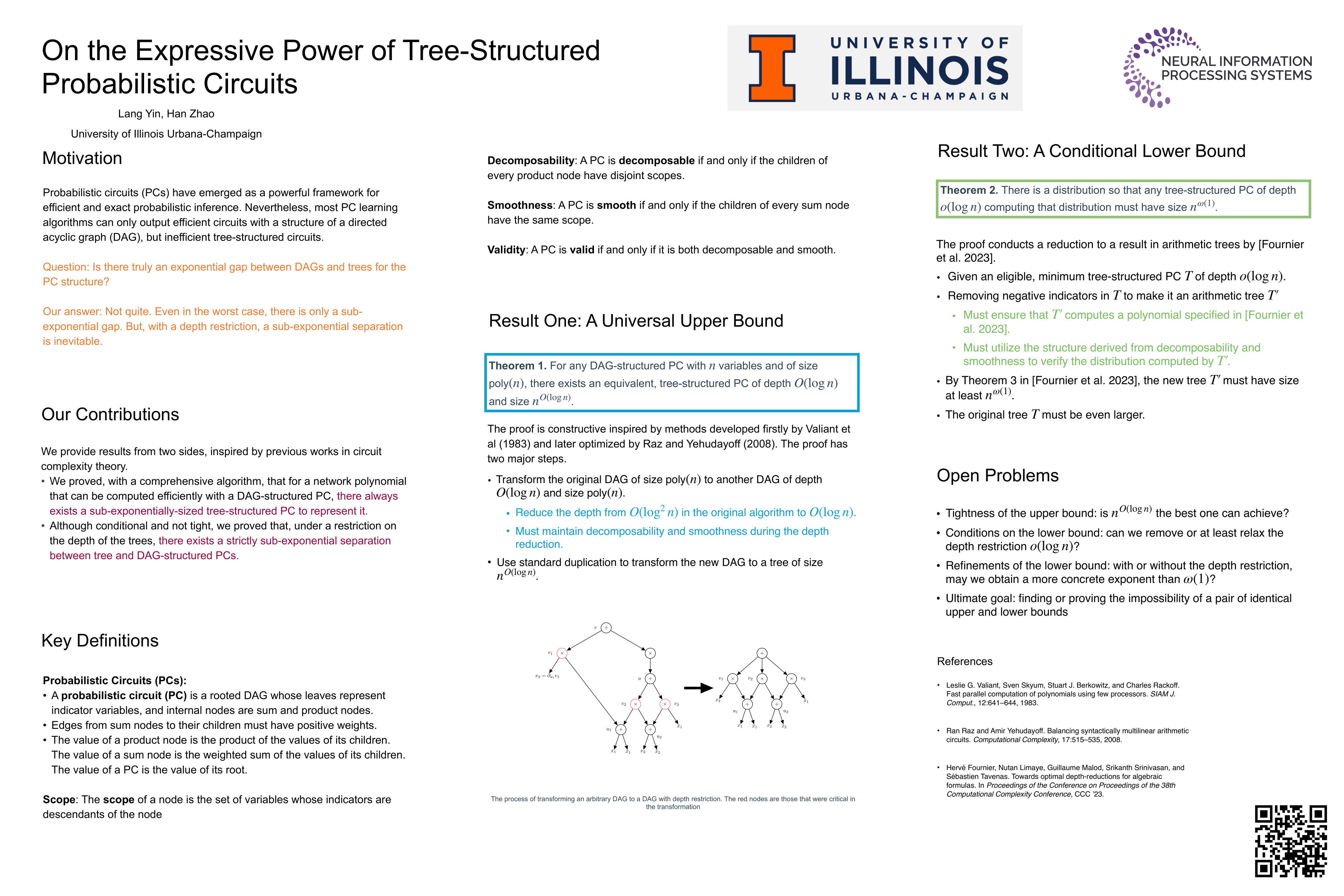
Abstract
Probabilistic circuits (PCs) have emerged as a powerful framework compactly representing probability distributions for efficient and exact probabilistic inference. It has been shown that PCs with general directed acyclic graph (DAG) structure can be understood as a mixture of exponentially (in its height) many components, each of which is a product distributions over univariate marginals. However, existing structure learning algorithms for PCs often generate tree-structured circuits, or using tree-structured circuits as intermediate steps to compress them into DAG-structured circuits. This leads to an intriguing question on whether there exists an exponential gap between DAGs and trees for the PC structure.In this paper, we provide a negative answer to this conjecture by proving that, for $n$ variables, there is a quasi-polynomial upper bound $n^{O(\log n)}$ on the size of an equivalent tree computing the same probability distribution. On the other hand, we will also show that given a depth restriction on the tree, there is a super-polynomial separation between tree and DAG-structured PCs. Our work takes an important step towards understanding the expressive power of tree-structured PCs, and our techniques may be of independent interest in the study of structure learning algorithms for PCs.